- Topic: Understanding Dockless Shared E-Scooter Travel Behavior: A Data-Driven Approach to Trip Purpose Classification
- Conference: 2025 Suwon ITS AP Forum
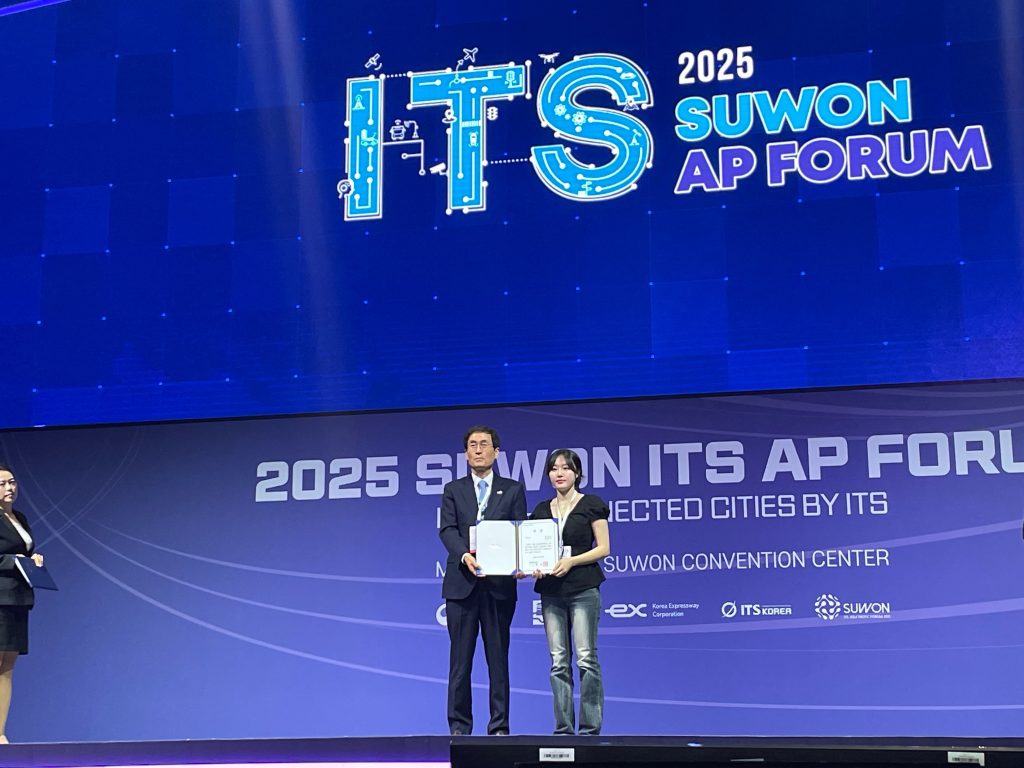
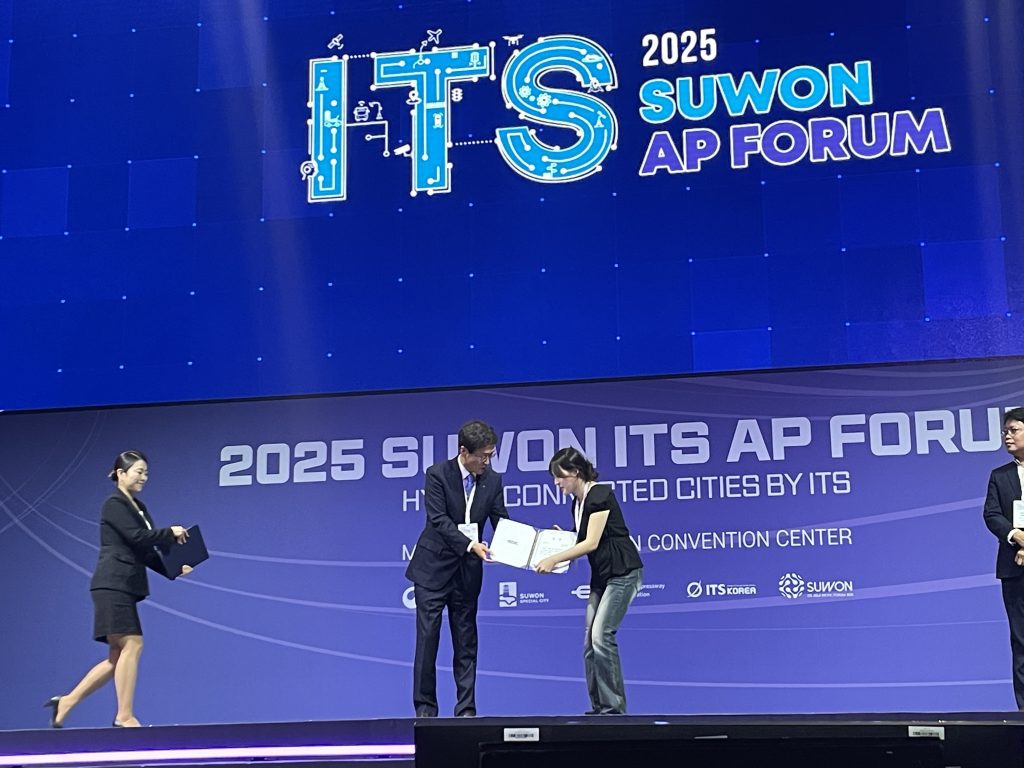
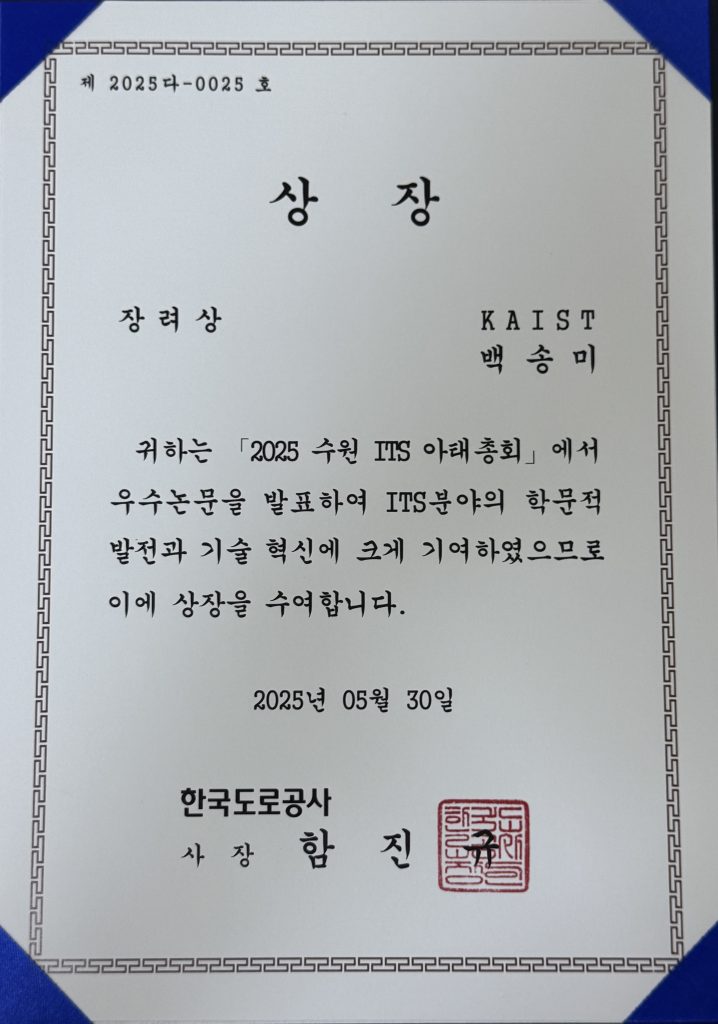
Unity-Vissim Cosimulation environment development for Human-in-the-loop test
Networking based Multiagent driving simulator platform development
KAIST Munji campus testbed transplantation to the virtual world
Traffic volume imputation based on multi-source data collection system
→ The overall multi-source data aggregation process and imputation procedure
A deep spatio-temporal approach in maritime accident prediction
– Predicting the risk of maritime accidents is crucial for improving traffic surveillance and marine safety.
→ The proposed DSTOAP architecture and prediction results for each grid size
Interactive driving behavior in overtaking situation
Human Recognition based on ITS technology
Pedestrian behavior
Optimized Signal plan development
This research is to integrate communication, traffic and driver behaviours, vehicle dynamics, and environment conditions in a unison framework to increase safety, reliability, and performance of connected and automated vehicles within a mix traffic condition.
To achieve this goal, TUPA has been developing an architecture that leverages existing tools like VISSIM, Driving simulator, Virtual Reality and Python to analyze and simulate multiple aspects of traffic including vehicles and pedestrians. This tool will enable to develop and validate techniques to improve traffic congestion, safety, and network performance.
In designing scenarios for the integration of Vissim and Driving simulator, a robust algorithm has been successfully implemented in the platform. This algorithm adapts its behaviour (traffic flow in transport) autonomously in response to the variation of network conditions.
Having human in the loop setting, alternative route guidance information will be displayed in driving simulator so that driver’s decision if he or she is willing to change the route based on the information will be recorded. In this process, the subject vehicle in the simulator and surrounding traffic in VISSIM will interact each other. In addition, all recoded outcomes from the driving simulator will be input to traffic simulation for the large network evaluation. The developed traffic simulation will identify typical traffic indicators such as delay and extreme delay, queue and the number of stops based on the scenarios developed in the driving simulator.
This research keeps developing with the KAIST team since middle of 2019. Transportation experts are aware that it is urgent to take measures to cope with mixed traffic between autonomous vehicles and conventional vehicles, which will be indispensable in the future. It is expected that this new traffic flow not only directly and indirectly leads to traffic accidents but also has a serious side effect on traffic efficiency. Until a fully autonomous vehicle occupies the road, new traffic control techniques are needed. In this study, we propose a new traffic signal controller that supports autonomous driving by driving the driver safely through the virtual environment. This study is expected to be synergistic effect of performance evaluation for future traffic in conjunction with the Connected ITS project and the K-City autonomous vehicle test bed currently underway in Korea. It is expected that many universities and research institutes will benefit from this research since Korea has not developed a human participatory machine learning platform using virtual reality or is in the very early stage.
The dashboard below was developed through Elastic open source software using the Seoul metro passenger flow data in 2014.
Data visualization has been important in democratizing data and analytics and making data-driven insights available to workers throughout an organization. Data visualization also plays an important role in big data and advanced analytics projects. As a transportation field accumulated massive troves of data during the early years of the big data trend, they needed a way to quickly and easily get an overview of their data. Visualization tools were a natural fit.
Visualization is central to advanced analytics for similar reasons. When advanced predictive analytics or machine learning algorithms are available, it becomes important to visualize the outputs to monitor results and ensure that models are performing as intended. This is because visualizations of complex algorithms are generally easier to interpret than numerical outputs.
In TUPA, we utilized one of the strongest searching engines called Elasticsearch to make this visualization works. The flowchart below shows the process to deal with big data and visualize it.
For more detailed information please contact our TUPA members below;
Xu Yanping, [email protected]
Cheng Lyu, [email protected]
We utilized TurtleBot3 which adopts ROBOTIS smart actuator Dynamixel for driving.
TurtleBot3 is a ROS-based mobile robot. We customized it to reconstruct the mechanical parts and use optional parts such as the computer and sensor. SLAM, Navigation and Manipulation, makes it to build a map and can drive around the room. Also, it can be controlled remotely from a laptop, joypad or Android-based smart phone.
The project allows the robot to detect the lane(s) and obstacles to avoid. Various algorithms such as SLAM, CNN, LSTM and OpenManipulator are embedded in the robot to make better robot behavior.
The following video demonstrates the navigation function.
For more detailed information please contact our TUPA members below;
Taeho Oh, [email protected]
Xu Bicheng, [email protected]
To provide empirical evidence on the relationship between built environment and public sharing bike flow in Suzhou, China.
The study area of this research focuses on Suzhou located in the southeast Jiangsu Province of East China and east about 100 km to Shanghai (Figure 1(A)).
There are around 1,750 bike stations and 40,000 public sharing bikes put into use in Suzhou (Figure 1(B)).
Fig. 1: Study area. (A) Location of Suzhou in China; and (B) the spatial distribution of bike stations, metro stations and population density in urban area of Suzhou.
Data Sources
Global Regression
Geographically Weighted Regression (GWR)
GWR is a local regression model. Coefficients are allowed to vary.
Bi-squared Weighting Function
Global Regression
Table 1: The results of Global Regression.
Dependent variables | Global Regression | |
Trips on workdays | Trips on nonwork days | |
Coeff. (t-value) | Coeff. (t-value) | |
Intercept | -75.388 (-8.27) | -67.948 (-7.25) |
Attributes of public bike systems | ||
Capacity of bike stations | 3.508 (11.88) | 3.372 (11.11) |
Accessibility to bike stations | 33.441 (14.66) | 27.991 (11.93) |
Built environment | ||
Population density | -2.2E-04 (-2.13) | -2.2E-04 (-2.00) |
Accessibility to metro station | 4.852 (5.83) | 3.803 (4.44) |
Accessibility to shopping mall | 8.254 (4.23) | 10.003 (4.99) |
Accessibility to bus station | 6.719 (3.23) | 6.054 (2.83) |
Accessibility to restaurant | 1.075 (5.82) | 1.491 (7.84) |
Accessibility to dwelling | 0.544 (0.49) | 0.411 (0.36) |
Accessibility to local financial services | 11.608 (6.84) | 11.494 (6.59) |
Accessibility to public leisure and religion place | -3.258 (-2.22) | -0.906 (-0.60) |
Accessibility to public park | -7.613 (-1.22) | -1.131 (-0.18) |
Accessibility to educational place | 7.276 (3.83) | 9.130 (4.68) |
Accessibility to workplace | 5.190 (3.78) | -1.610 (-1.14) |
R-square | 0.392 | 0.360 |
Adjusted R-square | 0.387 | 0.355 |
Note: Values in bold are significant at 0.1 level.
Fig. 3: Comparisons of explanatory power of Global regression and GWR.
Fig. 4: Spatial distributions of local coefficients on working day and t-value with significance less than 90%.
Global Regression |
GWR |
The capacity and proximity of bike stations are positively correlated with bike usage. Gravity-based accessibility to metro stations of bike stations may increase bike flow. The bike stations nearby shopping malls, bus stations, restaurants, financial and educational places are also positively correlated with bike usage. Population density has a statistically negative impact on bike usage. |
The effects of built environment are divergent across the Suzhou region. Most of the coefficient appears to have zero or negative value in the central areas of Suzhou (Old Town) while surrounding areas have modest built environment effect on bike flows. |
The goodness of fit in the GWR is better than the global regression model.
This work was supported by Jiangsu Industrial Technology Research Institute and Research Institute of Future Cities at Xi’an Jiaotong-Liverpool University.
For more detailed information please contact our TUPA members below;
Chunliang Wu, [email protected]
Transportation data is of great importance for intelligent transportation system. Missing data problems are inevitable during data collection.
Challenges in existing imputation methods: potential useful information is not efficiently used in the modeling process; methods considering temporal correlation usually assuming that linear relationships exist between observed variables and latent variables; most techniques fail to measure the uncertainty.
This study introduces the use of a self-measuring multi-task Gaussian process (SM-MTGP) method for imputing missing data.
A SM-MTGP method is proposed to combine features from tasks and inputs to measure similarities jointly.
Dependencies of tasks and inputs are explored via covariance functions under SM-MTGP framework.
Correlations between responses are captured to provide additional information for enhancing imputation accuracy.
Brief review of MTGP
Assuming we have \(Q\) tasks and a set of observations \(Y = \left\{ {{y_{i1}},{y_{i2}}, \ldots {y_{iD}}} \right\}, i = 1, \ldots ,Q\), for each corresponding task at \(????\) distinct inputs, where \(????_{????????}\) is the response for \(????^{????ℎ}\) task given the input \(????_????\).
FIGURE 1 Vectorization of matrix Y
When the SM-MTGP model is introduced to the imputation of missing values of transfer passenger flow, the shared information of tasks is considered in terms of the temporal relatedness of various days. Transfer passenger flow over \(Q\) days can be treated as \(Q\) tasks, and the number of sampling time intervals \(D\) per day represents \(D\) distinct inputs. We define a matrix \(Y = \left\{ {{y_{ij}}} \right\}(i = 1,2,…,Q;j = 1,2,…D) \in Q \times D \), where \({{y_{ij}}}\) is number of transfer passengers for the \({i^{th}}\) day (task) on the \({j^{th}}\) time interval (input). By stacking the column vectors of \(Y \in Q \times D \), a \(Q \times D\) dimension vector \({\bf{y}} = vec(Y)\) is obtained (Figure 1).
The MTGP model of \({{\bf{\tilde y}}}\)can be described as Equation (1):
$${{\tilde y}_{ij}} = {m_{ij}} + \varepsilon ,\quad \varepsilon \sim N\left( {0,{\sigma ^2}} \right) \tag{1}$$ where \({m_{ij}}\) is the expected value of the element \({{\tilde y}_{ij}}\), and \(\varepsilon\) is an additive Gaussian noise with variance \({{\sigma ^2}}\).
$$m \sim N\left( {0,{\Sigma _Q} \otimes {\Sigma _D}} \right) \label{TGP} \tag{2}$$ $${\Sigma _Q} = K_Q^fG_Q^m, \quad{\Sigma _D} = K_D^fG_D^m \label{covariance matrix} \tag{3}$$ The covariance matrices \({\Sigma _Q}\) are defined as a product of kernel of days features (tasks) \(K_Q^f\) and the self-measuring kernel \(G_Q^m\), and \({\Sigma _D}\) are defined as a product of the kernel of time intervals features (inputs) \(K_D^f\) and self-measuring kernel \(G_D^m\).
$${K_Q^f} = k\left( {{y_i},{y_j}} \right) \in \mathbb{R}^{Q \times Q}, \quad {G_Q^m} = g\left( {{y_{i:}},{y_{j:}}} \right) \in \mathbb{R}^{Q \times Q} \tag{4}$$ $${K_D^f} = k\left( {{y_h},{y_l}} \right) \in \mathbb{R}^{D \times D}, \quad {G_D^m} = g\left( {{y_{:h}},{y_{:l}}} \right) \in \mathbb{R}^{D \times D} \tag{5}$$ where \(k\left( {{y_i},{y_j}} \right)\) and \(k\left( {{y_h},{y_l}} \right)\) indicate covariances of features of \({i^{th}}\) day and \({j^{th}}\) day, and covariances of features of \({h^{th}}\) time interval and \({l^{th}}\) time interval, respectively. Similarly, \(g\left( {{y_{i:}},{y_{j:}}} \right)\) and \(g\left( {{y_{:h}},{y_{:l}}} \right)\) measure covariances of self-measuring observations of \({i^{th}}\) day and \({j^{th}}\) day, and covariances of self-measuring observations of \({h^{th}}\) time interval and \({l^{th}}\) time interval.
By following the principle of MTGP, the joint distribution of \({\tilde Y}\) can be described as Equation (6), where \(\Phi = {\Sigma _Q} \otimes {\Sigma _D} + {\sigma ^2}{\bf{I}}\).
$$\int {p\left( {\tilde Y|M,0,{\sigma ^2}} \right)} p\left( {M|{\Sigma _Q},{\Sigma _D}} \right)dM = N\left( {{\bf{\tilde y}}|{\bf{0}},\Phi } \right) \tag{6}$$ Using a Gussian process framework given the observed number of transfer passengers, the unobserved passenger flows in \(Y\) can be derived by predictive equation (7). $$E[{{\tilde y}_{ab}}|{{{\bf{\tilde y}}}_{obs}},{\Sigma _Q},{\Sigma _D}] = \left( {{\Sigma _{{Q_a}}} \otimes {K_{{D_b}}}} \right)_{obs}^T\Phi _{obs}^{ – 1}{{{\bf{\tilde y}}}_{obs}} \tag{7}$$ where \({\Phi _{obs}} = {\bf{P}}\Phi {{\bf{P}}^T} \in \mathbb{R}^{M \times M} \) is a covariance matrix over the observed transfer passenger flows in \(Y\), \({{\Sigma _{{Q_a}}}}\) denotes \({a^{th}}\) column vector in \({\Sigma _Q}\), which measures the similarities between \({a^{th}}\) day and all the other days among \(Q\) days, and \({{K_{{D_b}}}}\) indicates \({b^{th}}\) column vector of \({K_D}\), which represents covariance between \({b^{th}}\) time interval and all the remaining time intervals of \(D\) samples.
Data analysed includes 6-months of passenger flow data collected by WiFi sensors at Richmond railway station (Figure 2), Melbourne, Australia.
FIGURE 2 Map of location and train lines of Richmond station.
FIGURE 3 Map of 12 WiFi sensors distribution.
The deployed 12 sensors are distributed at platforms 7-10 and two sided underpasses (Figure 3).
Figure 4 indicates the RMSE results of discrete missing pattern with various algorithms. The improvements in RMSE by SM-MTGP is around 60% for three different missing rates.
FIGURE 4 RMSE Results for Different Missing Ratios of Discrete missing data.
Three mixed missing patterns under different missing ratios are reported (Figure 5-7). The SM-MTGP method is still able to obtain better performance compared with all the other methods, leading to improvements in RMSE up to 60%.
FIGURE 5 RMSE Results for Different Missing Ratios of Mixed Missing Data
with One Random Day Missing.
FIGURE 6 RMSE Results for Different Missing Ratios of Mixed Missing Data
with Two Random Day Missing.
FIGURE 7 RMSE Results for Different Missing Ratios of Mixed Missing Data
with Four Random Day Missing.
Imputation accuracy can achieve around 60% improvement in RMSE in all the tested missing scenarios compared with the base model.
SM-MTGP significantly outperforms other methods under the large missing ratio.
On-going research on incorporating other features into this algorithm to make application on large-scale transit network and simplifying model computational complexity.
For more detailed information please contact our TUPA members below;
Wenhua Jiang, [email protected]
© 2025 TUPA. All rights reserved.